How-to Guide: Implementing Innovative Scientific Models for Technology Development
Understanding the Importance of Innovative Scientific Models
In the rapidly evolving landscape of technology development, staying ahead of the curve requires more than just keeping up with current trends. It demands the implementation of innovative scientific models that can drive new advancements and solutions. These models offer a framework for understanding complex systems and predicting outcomes, which are crucial for making informed decisions in the tech industry.
Scientific models help break down abstract concepts into manageable components, making it easier to explore new possibilities. By leveraging these models, technology developers can enhance their ability to innovate, optimize processes, and ultimately create products and services that meet the demands of a dynamic market.
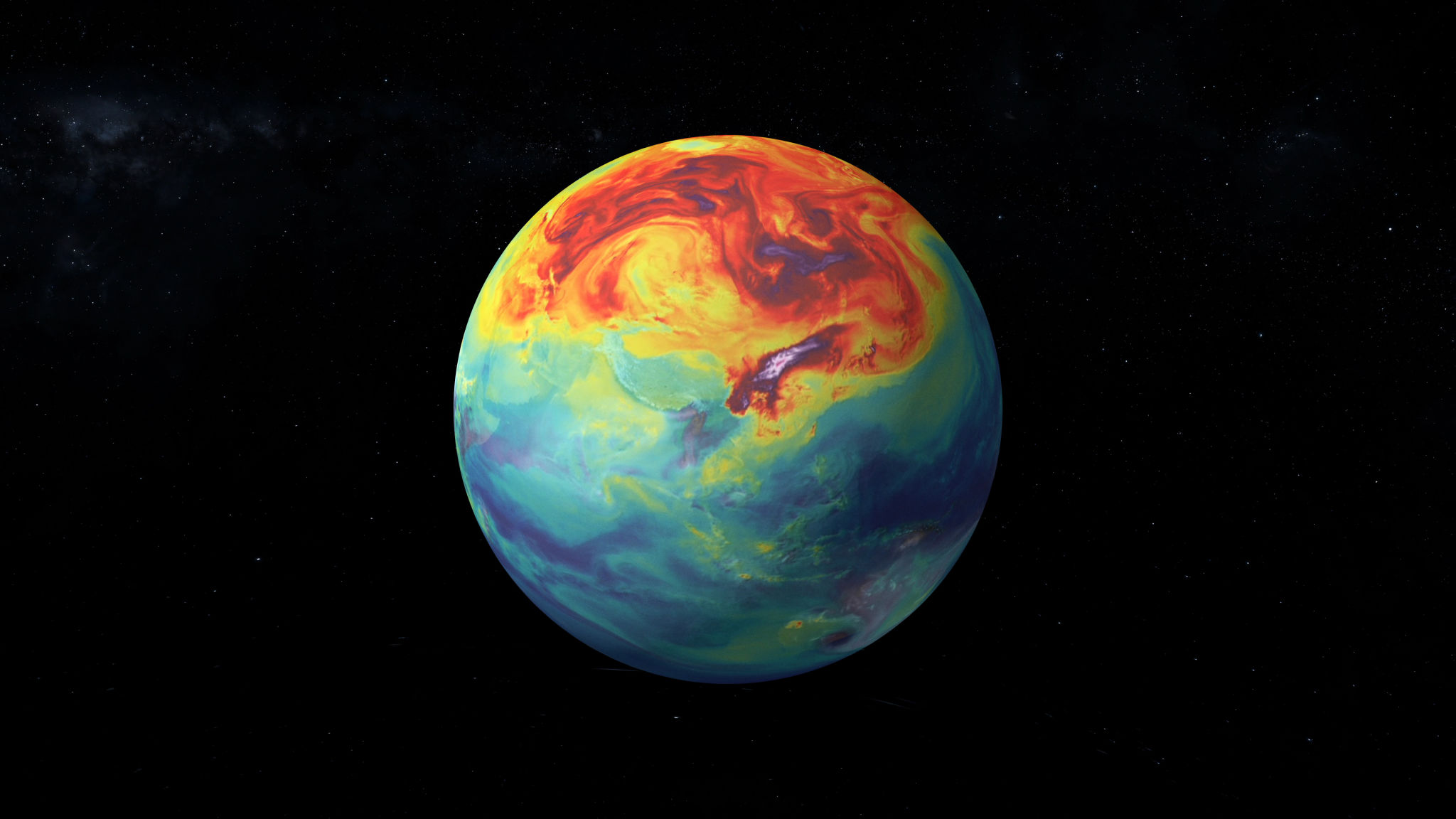
Identifying the Right Scientific Model for Your Project
Choosing the appropriate scientific model is a critical step in the development process. Not all models are created equal, and selecting one that aligns with your project's goals is essential. Begin by evaluating your project's specific needs, challenges, and desired outcomes. This evaluation will guide you in identifying a model that offers the best fit.
Some common types of scientific models used in technology development include mathematical models, simulation models, and data-driven models. Each type has its strengths and applications. For instance, mathematical models are excellent for projects requiring precise calculations, while simulation models are ideal for visualizing complex systems.
Mathematical Models
Mathematical models use equations and formulas to represent real-world systems. They are particularly useful in scenarios where precision and quantitative analysis are crucial. These models can predict future trends, optimize processes, and provide insights into system behavior under various conditions.
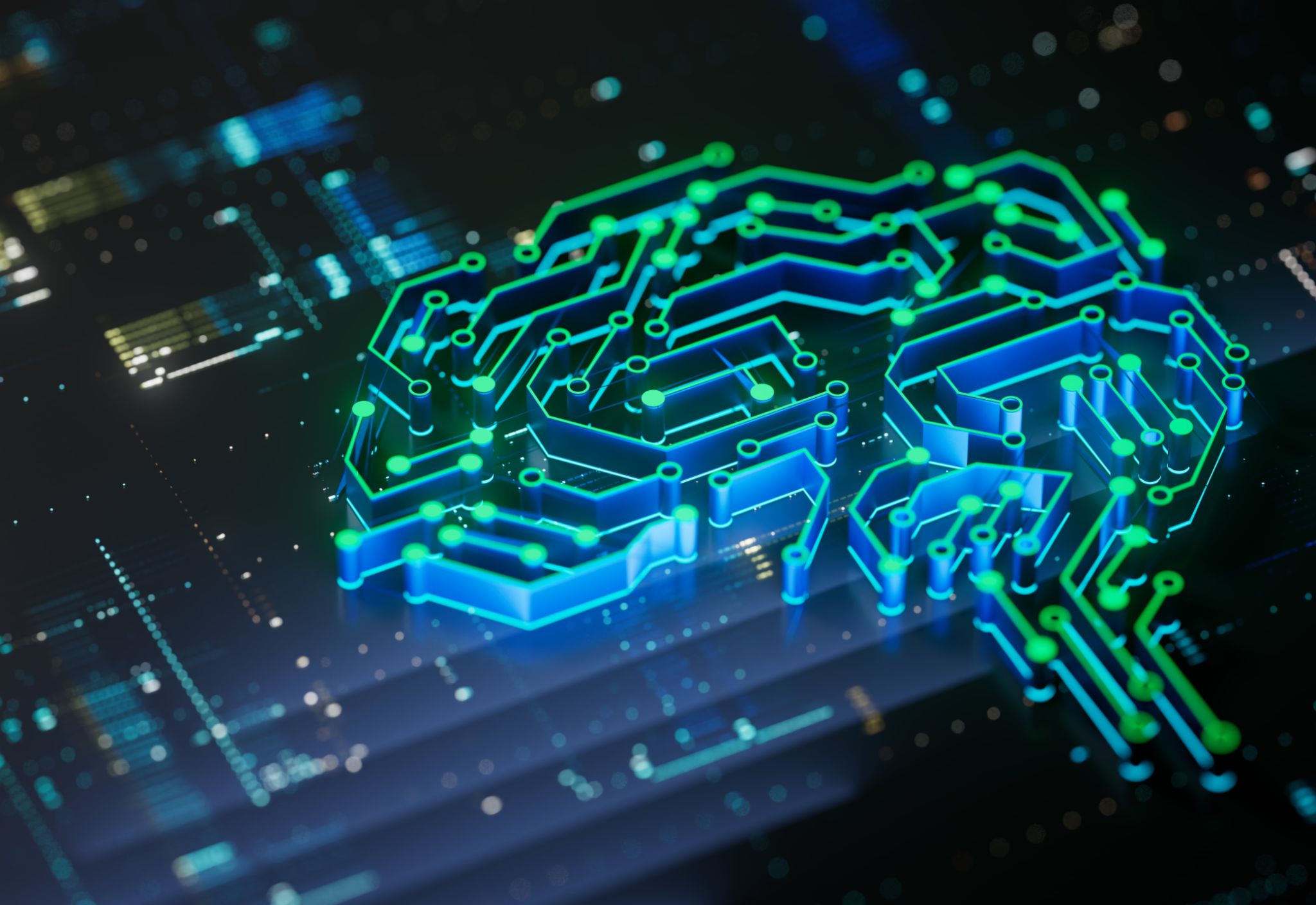
Simulation Models
Simulation models allow developers to create virtual representations of systems to test different scenarios. This approach is advantageous for understanding how changes to a system will impact outcomes without the risk or cost associated with physical trials. Simulation models are commonly used in fields like aerospace, automotive design, and software development.
Integrating Data-Driven Models
In today's data-rich environment, data-driven models have gained prominence in technology development. These models leverage large datasets to uncover patterns, make predictions, and inform decision-making processes. By integrating machine learning and artificial intelligence, data-driven models can continuously improve as more data becomes available.

Implementing data-driven models requires careful attention to data quality and relevance. Ensuring that your dataset is comprehensive and representative is key to achieving accurate and meaningful insights. Additionally, developers should consider ethical implications and transparency when using data-driven approaches.
Overcoming Challenges in Model Implementation
While scientific models offer numerous benefits, their implementation is not without challenges. Common obstacles include data limitations, computational constraints, and model complexity. Overcoming these challenges often requires interdisciplinary collaboration, where experts from various fields work together to refine and enhance model accuracy.
To successfully implement innovative scientific models, it is essential to foster a culture of continuous learning and experimentation within your organization. Encouraging team members to explore new methodologies and stay updated on the latest research can lead to breakthroughs in technology development.
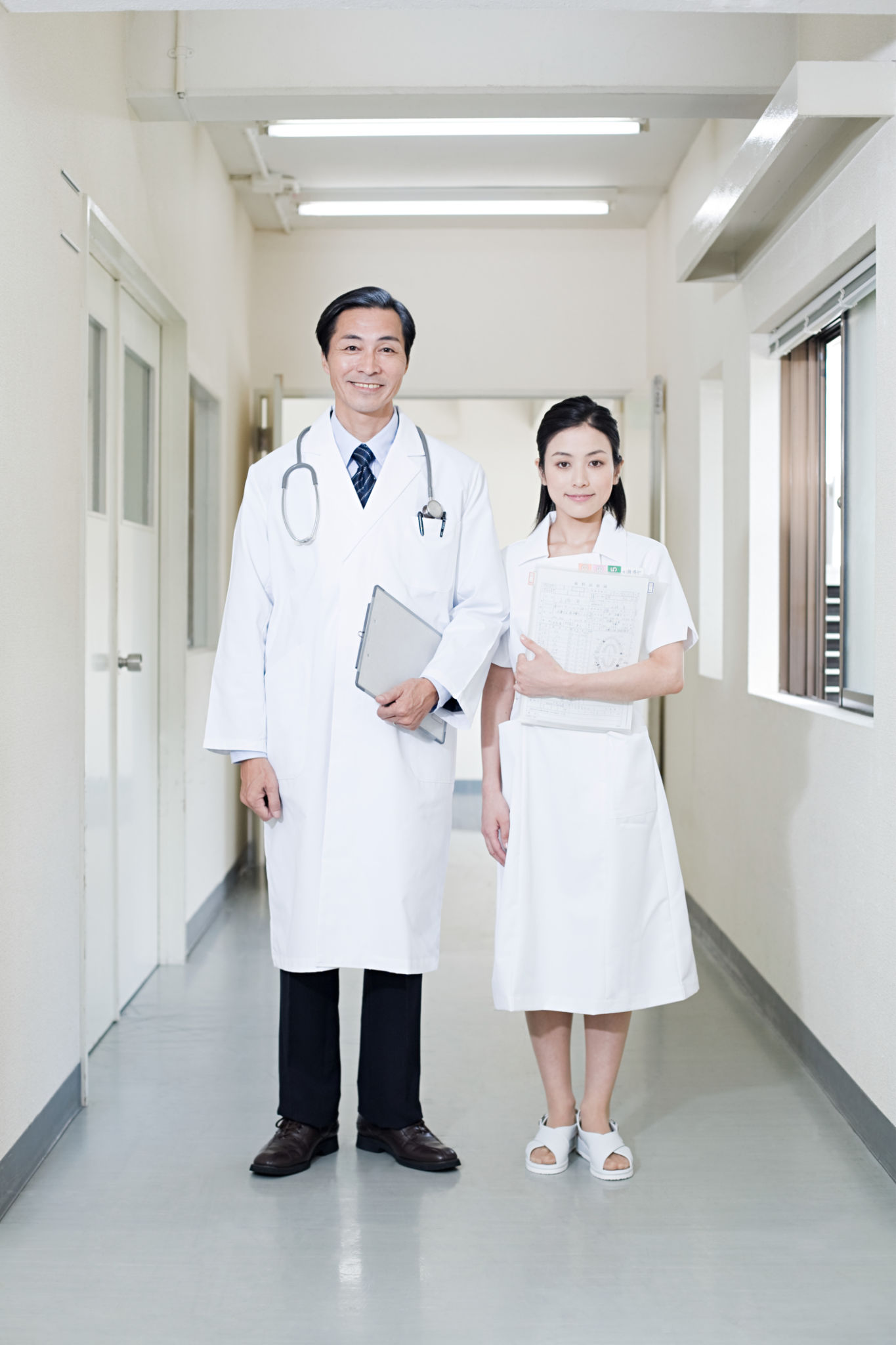
Conclusion: Embracing Innovation for Future Success
The integration of innovative scientific models into technology development is a transformative approach that can propel organizations toward success. By understanding the nuances of different model types and addressing implementation challenges, developers can harness the power of these tools to create cutting-edge technologies.
As the tech industry continues to evolve, embracing innovation through scientific modeling will be key to staying competitive and responsive to market needs. By investing in these methodologies today, organizations can build a foundation for future growth and innovation.